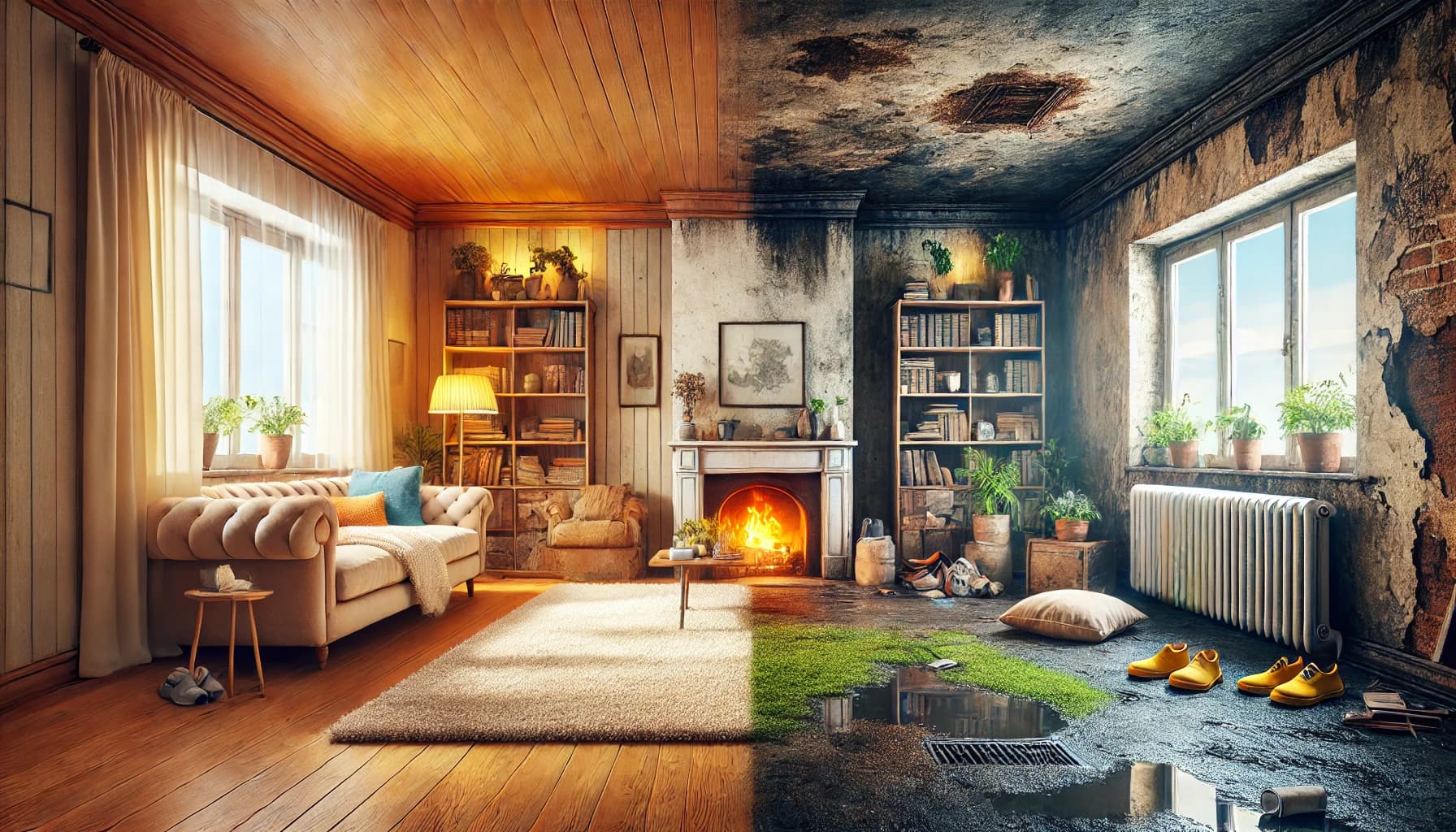
Beyond the Numbers: The Limitations of A/B Testing
User Experience (UX) has become a bit of a hot topic when it comes to the design of any digital product. While there does seem to be a genuine interest in improving user experiences, there is often a misalignment between the want for good UX and the value placed on user research.
Some businesses want a quick fix to user experience based on so-called ‘best practices’, and attempt to skip the user research phase altogether. Or, they want a catch-all UX research method that will allow them to check the ‘user research’ box.
This is when the words ‘A/B testing’ tend to get thrown around.
A Quick Fix for User Experience
From the outside, the concept of A/B testing is simple: test two versions of a webpage, email, or other digital assets with live users to see which performs better. Very often, it’s a test between the existing design and a new, updated design.
At the end of this test, you have the numbers to show which design was more successful in helping users achieve the goal that we had in mind for them.
But is it really that simple? If it was… this would be a very short blog post.
When used appropriately, A/B testing can offer valuable insights. However, it’s far from a one-size-fits-all solution. In fact, A/B testing can often lead to skewed or misleading results if not approached with caution.
A Closer Look at A/B Testing
A successful A/B test requires more than just setting up two versions and waiting for results to roll in. Far from being an isolated experiment, A/B testing demands a comprehensive research plan, to ensure that the insights you gather are accurate and actionable.
It starts with clear objectives — defining what you’re trying to achieve and what success looks like. The next step involves crafting a solid hypothesis: a well-informed assumption about how a specific change might impact user behaviour.
Once the goals and hypotheses are set, thorough planning is essential to determine the right user segments, traffic distribution, and metrics to track. Proper preparation also involves considering seasonality, and user context, and avoiding external factors that could skew results.
Only after this groundwork is done can the actual A/B testing begin.
But, how can we do the groundwork properly if no other research has been done? Well… you can’t.
If you try to, you are very likely to run into some very common pitfalls of A/B testing.
Correlation vs. Causation: A Common Trap
A fundamental flaw in interpreting A/B test results is confusing correlation with causation.
Just because a change correlates with improved performance doesn’t mean the change caused the improvement.
Many factors could cause one option to perform better than the other in an A/B test. These factors have nothing to do with the ‘winning’ option being better designed or more user-centric, such as user behaviour, seasonality, user segmentation, insufficient sample sizes and baseline bias.
A/B Testing Scenario
Before we dive into those influencing factors, imagine this scenario…
In early November, a toy store website redesigned its landing page with the goal of driving more conversions. They want to launch the new landing page by April of the following year. No user research was done prior to the redesign, it was based on stakeholder input and assumptions.
The company doesn’t want to launch the new page without having done some sort of research to ensure that it will be successful, but they don’t have the budget for interviews or usability testing. For this reason, someone on the team suggested A/B testing of the current landing page vs. the new one, to see which performs better.
Looking at their analytics from years previous, they can see that in early November, there is generally a huge surge in traffic, but no increase in sales, and occasionally a decrease in sales. Then in the second half of November, sales skyrocket, and the high conversion rate continues into December and starts to ease up in mid-late January.
Let’s look at how this test could be influenced by user behaviour, sample group size, segmentation and baseline bias.
User behaviour
User behaviour is inherently complex, and context plays a massive role in how a user interacts with a digital product. Factors such as context and seasonality can hugely influence user behaviour and can be the difference between a conversion and a drop-off.
In our toy-store example, seasonality, more specifically Christmas, is undoubtedly affecting user behaviours.
The site sees an increase in traffic as users are browsing in early November and an increase in conversions as the Black Friday sales happen and the deadline to buy Christmas presents looms ever nearer.
If we were to run A/B testing at this time, that festive anticipation and increased traffic would heavily influence the outcome, leading to skewed insights.
During this high-traffic period, user behaviour is not representative of a typical shopping experience in April, when the new landing page is due to be launched. Therefore any insights into performance may be misleading and could lead to some very disappointed stakeholders when the new version launches and they don’t see anywhere near the number of conversions seen in the testing.
Insufficient sample sizes and poor user segmentation
Similarly, insufficient sample sizes and a lack of proper user segmentation can make A/B test results inconclusive or misleading. In the toy store example, testing with a small group of users might fail to capture the diversity of your audience. And, not segmenting users effectively can lead to biased conclusions.
The user groups for the toy store website will be pretty diverse. Each user group will have very different behaviours on the site. For example, a chunk of the users might be children, just looking at what’s available and showing their parents or making wishlists. There will be adult users, who are on a buying mission, with an exact product(s) in mind. There will also be users who are just browsing with the intent to buy at a later date, online or in-store.
If we just run an A/B test on this group of users, without any segmentation, we risk having unbalanced user groups testing each variant. I.e. If the group that sees option A is primarily children and the group that sees option B is their parents, then option B will naturally perform better in terms of conversions. But that result will have little to do with it being a better design or experience.
Baseline Bias
A/B testing of a current version of a site against a new design comes with its own issues. It assumes that the current version of a site is the optimal baseline to test against, which can distort results.
If the current design is flawed, any new version will seem like an improvement, even if it’s not the best solution. This can lead to overconfidence in the new design simply because it surpasses a low benchmark.
Beyond A/B Testing: A Holistic Approach to UX Research
A/B testing certainly has its place in the UX toolkit, but it shouldn’t stand alone. Pairing it with other research methods can provide a more rounded understanding of user needs and behaviours.
Qualitative testing methods, like moderated user testing, can offer a depth of insight that A/B tests can’t. In moderated sessions, real users are asked to perform tasks and share their thoughts and feedback. Unlike A/B testing, this method allows you to observe the “why” behind user actions, uncovering motivations and pain points that raw data might not reveal.
Combining qualitative research with A/B testing creates a powerful blend of data: the numbers tell you what’s happening, and the user feedback explains why it’s happening. This balance helps to validate assumptions, refine testing hypotheses, and ultimately design better user experiences.
Conclusion: A Balanced Strategy for Reliable Insights
The most effective UX strategies involve combining both quantitive research, like A/B testing and qualitative research like usability testing. A/B testing gives you clear numbers that show what’s working and usability testing provides the “why” behind those numbers. Together, they create a fuller picture of user behaviour.
Before diving into A/B testing, it’s crucial to validate assumptions through user research. Continuous feedback throughout the design process can pinpoint areas for improvement before the tests even begin. This layered approach ensures that A/B testing isn’t a shot in the dark but part of a broader, informed strategy.
In the end, A/B testing is a valuable tool, but it’s just one piece of the UX puzzle. For a truly effective user experience, it’s essential to look beyond the numbers and understand the people behind them.
If you’re interested in conducting a well-rounded user study, get in touch with Friday to find out how we can develop a process to meet your specific needs.